In the never-ending arms race between threat actors and defenders, automation and machine learning have become your ultimate weapons. Today, threat actors employ automation in countless ways to speed up their attacks and evade detection. Outpacing attackers requires the effective use of automation and machine learning.
Years ago, our research and development teams recognized it wasn’t possible to stay ahead of attackers with only human-led research and analysis techniques. So, we made it our mission to automate every possible aspect of attack detection and enforcement that we could. This relentless drive toward automation allowed us to analyze content and update our defenses faster than attacks could spread.
When we introduced WildFire cloud-based malware prevention service in 2011, we not only automated file collection and analysis, we also accelerated time-to-protection by quickly distributing new protections to our global community of customers. With WildFire, customers could stay ahead of fast evolving malware with shared protections and zero operations impact.
WildFire continued to evolve, and it now employs a suite of advanced analysis techniques to uncover stealthy zero-day threats, including dynamic, static, and bare-metal analysis. Each type of analysis involves multiple steps, examining a variety of different behaviors and attributes to uncover the most advanced threats. For example, WildFire’s static analysis engine uses supervised and unsupervised machine learning to detect new malware families. Our supervised machine learning models look at hundreds of file attributes, including file size, header information, entropy, functions, and much more to train a machine learning model to identify the most novel malware.
Staying ahead of quickly changing malware requires constantly updating detection algorithms based on new data. Machine learning is the only practical way to analyze massive volumes of malware artifacts quickly, as human analysis simply cannot scale against this volume. To date, WildFire has processed billions of samples and identified trillions of artifacts. This vast amount of data improves our ability to distinguish malware from legitimate files.
Daily Samples by Filetype
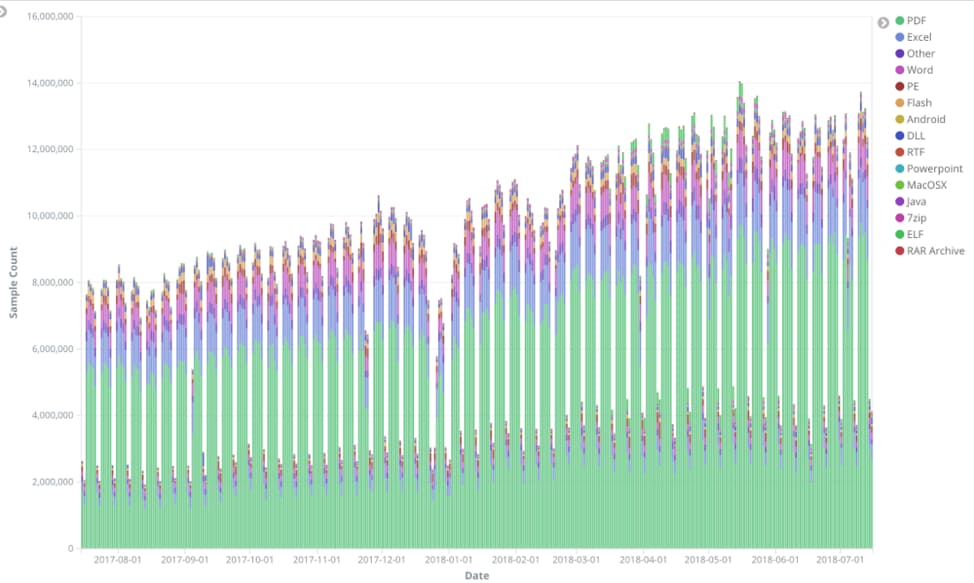
WildFire analyzes millions of unknown samples every month.
One of the techniques WildFire uses to detect malware is byte code analysis. When WildFire receives a new, unknown file, it builds a histogram of byte character frequency and compares this histogram to patterns from known malware families.
To dive deeper, WildFire uses a random forest algorithm to analyze byte code distributions. Random forest classification focuses on certain, high-yield byte patterns while ignoring byte patterns with noisy data. This statistical fingerprint enables WildFire to detect polymorphic variants of known malware that can evade traditional signatures. WildFire’s static, dynamic, and bare-metal analysis engines complement one another; each technique can be trained on datasets that evade the other, resulting in extremely accurate attack detection.
Machine learning is not just essential for malware analysis. It can be applied to many aspects of security to detect never-before-seen threats and increase the speed and scale of threat protection.
To learn how machine learning is used in security, register for our October 30 webinar “Machine Learning 101: Learn How to Streamline Security and Speed up Response Time.”